Recently, a lot has transpired in the world of Google Ads. This year, the keyword match type behavior witnessed a significant change, the Responsive Search Ad became the default ad type, and much more. Not to forget, Experiments, previously known as Drafts & Experiments, has undergone a shift as well. What the change is and how you should go about it, we discuss in this post.
What Is New?
Advertisers were formerly, required to create Drafts. With Drafts, they could mirror their campaign’s settings and make changes without impacting the original campaign performance. One could decide whether to test the changes (run an experiment) or apply them directly to the campaign. A draft run as an Experiment would test the impact of the changes that you intended to test and would compare its performance with the original campaign over time.
All the above is a thing of the past now! Advertisers are no longer required to create Campaign Drafts.
So what has replaced it?
Now, you can run tests on experiments on a new experiments page under the “All Experiments” section. Here you can create the following types of experiments:
- Custom Experiments
- Ad Variations
- Video Experiments
In this post, we will discuss the steps to create Custom Experiments. But before that let’s first get a quick understanding of what it is:
Custom experiments are typically used to test Smart Bidding, keyword match types, landing pages, audiences, and ad groups. Custom experiments are available for Search and Display campaigns
How To Set Up Custom Experiments
1. Log in to your Google Ads account. Click on Experiments + All Experiments at the left side panel. Click on the “plus” sign to start creating the experiments
2. Click on custom experiments + choose the campaign type and click on continue
3. You will get to the page where you have to begin the process of setting up the experiments. Write the appropriate experiment name, choose the campaign you want to experiment with and click on save and continue.
Note: Select the campaigns that do not have a shared budget and ensure that any campaign level conversion goals are manually copied over to the sample campaign after it is scheduled.
4. After setting up the experiment, you can make edits to the experiment if required. These changes can be made on the campaign and ad group levels.
5. You can schedule the campaign after making the desired changes. Select experiment goals (up to two) by selecting the metric and increase or decrease those metrics by selecting them in the parallel section.
6. You can split the budget as per the portion of your original campaign’s traffic and budget. Also, you can select either search based split or cookie-based split.
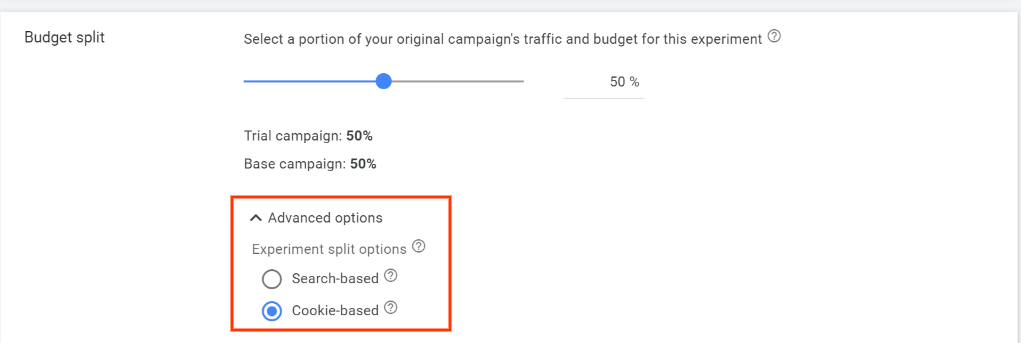
These advanced settings are intended to do the following:
Search based – With this option, every time a search happens, users get to either see the advertiser’s original campaign or the experiment randomly. Here there is a likelihood of the same user seeing both versions of your campaign. As clear, the aim of this experiment split option is to count the searches.
Cookie-based – With this option, users are assigned to a bucket and get to either see the experiment or the original campaign regardless of how many times they conduct a search. The aim of this experiment split option is to count the users.
7. Mention start and end dates for your experiment after splitting the budget wisely and sync your base and trial campaigns together by enabling synchronization.
8. Once you create the experiment, it is ready to run on the selected date.
Analyzing your Experiments Data
Ideally, whenever you launch an experiment, rest for the first 2 weeks as that is the learning period. It will ramp up later and give you actionable insights to make decisions on. Here is how the results will appear.
The base and trial are the campaign and experiment data respectively. You will see the data for the duration of the performance comparison. The Difference row displays the increase or decrease in the value of a specific metric. For example, in the above snapshot, the experiment contributed to an additional 1288 Impressions over and above the impression received by the campaign. The following row (Difference%) shows the percentage of increase and decrease.
The 95% confidence row displays the range of the performance difference. In the above-shared example, [+5% +11%] meant that the campaign has a 95% chance of receiving a 5% to 11% difference for the metric, should the changes be incorporated. The term “satisfactorily significant” refers to the likelihood that the experiment would perform similarly if converted into a campaign.
To conclude, for the above example, we should ideally run it for some more time to see the impact on conversions. Since right now it does not have any significant data to give conclusions on those metrics
Similarly, you could decide on the metric for which you want to see improvement. If it is to reach more audiences, then the impressions metric could help you make the final decision and thereof.
A quick way to check multiple experiments
If you need help with understanding multiple experiments, then we have just the right solution for you. Our Experiment Performance Report Script fetches and compares the performance of experiment campaigns and compares it with the performance of its base campaign
Related Links
Stop the wasted ad spend. Get more conversions from the same ad budget.
Our customers save over $16 Million per year on Google and Amazon Ads.